
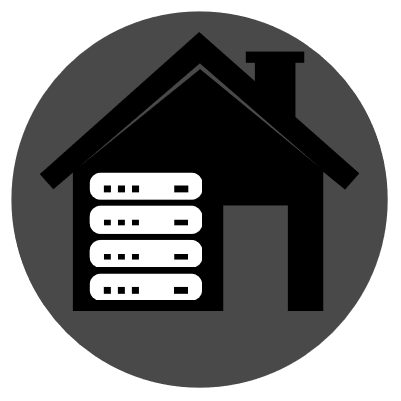
This is an extremely odd outlook to have. Good luck with it.
Unfortunately the answer to your question is to not post at all, though if your contributions are worthwhile then that is not an excellent solution
This is an extremely odd outlook to have. Good luck with it.
Unfortunately the answer to your question is to not post at all, though if your contributions are worthwhile then that is not an excellent solution
There’s is a huge difference though.
That being one is making hardware and the other is copying books into your training pipeline though
The copy occurs in the dataset preparation.
Privacy preserving federated learning is a thing - essentially you train a local model and send the weight updates back to Google rather than the data itself…but also it’s early days so who knows what vulnerabilities may exist
You could try dexed, it’s a YamahaDX7 clone https://github.com/asb2m10/dexed/releases
You need rebase instead. Merge just creates useless commits and makes the diffs harder to comprehend (all changes are shown at once, but with rebase you fix the conflicts in the commit where they happened)
Then instead of your branch of branch strat you just rebase daily into main and you’re golden when it comes time to PR
I don’t really follow your logic, how else would you propose to shape the audio that is not “just an effect”.
Your analogy to real life does not take into account that the audio source itself is moving, so their is an extra variable outside of just stereo signal -which is what spatial audio is modelling
And your muffling example sounds a bit over simplified maybe? My understanding is that the spatial stuff is produced by phase shifting the LR signals slightly
Finally why not go further? “I don’t listen to speaker audio because it’s all just effects and mirages to sound like a real sound, what only 2^16 discrete positions the diaphragm can be in” :p